DeepMind’s protein-folding AI has solved a 50-year-old grand challenge of biology
AlphaFold can predict the shape of proteins to within the width of an atom. The breakthrough will help scientists design drugs and understand disease.
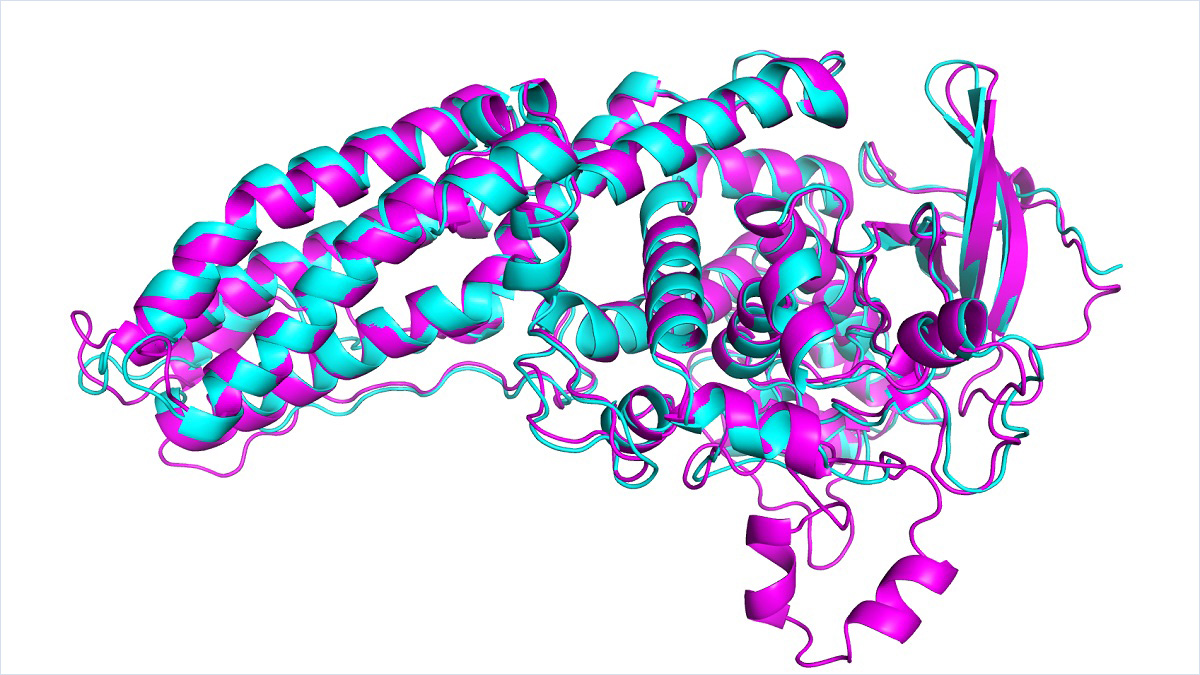
DeepMind has already notched up a streak of wins, showcasing AIs that have learned to play a variety of complex games with superhuman skill, from Go and StarCraft to Atari’s entire back catalogue. But Demis Hassabis, DeepMind’s public face and co-founder, has always stressed that these successes were just stepping stones towards a larger goal: AI that actually helps us understand the world.
Today DeepMind and the organizers of the long-running Critical Assessment of protein Structure Prediction (CASP) competition announced an AI that should have the huge impact that Hassabis has been after. The latest version of DeepMind’s AlphaFold, a deep-learning system that can accurately predict the structure of proteins to within the width of an atom, has cracked one of biology’s grand challenges. “It's the first use of AI to solve a serious problem,” says John Moult at the University of Maryland, who leads the team that runs CASP.
A protein is made from a ribbon of amino acids that folds itself up with many complex twists and turns and tangles. This structure determines what it does. And figuring out what proteins do is key to understanding the basic mechanisms of life, when it works and when it doesn’t. Efforts to develop vaccines for covid-19 have focused on the virus’s spike protein, for example. The way the coronavirus snags onto human cells depends on the shape of this protein and the shapes of the proteins on the outsides of those cells. The spike is just one protein among billions across all living things; there are tens of thousands of different types of protein inside the human body alone.
In this year’s CASP, AlphaFold predicted the structure of dozens of proteins with a margin of error of just 1.6 angstroms—that’s 0.16 nanometers, or atom-sized. This far outstrips all other computational methods and for the first time matches the accuracy of techniques used in the lab, such as cryo-electron microscopy, nuclear magnetic resonance and x-ray crystallography. These techniques are expensive and slow: it can take hundreds of thousands of dollars and years of trial and error for each protein. AlphaFold can find a protein’s shape in a few days.
The breakthrough could help researchers design new drugs and understand diseases. In the longer term, predicting protein structure will also help design synthetic proteins, such as enzymes that digest waste or produce biofuels. Researchers are also exploring ways to introduce synthetic proteins that will increase crop yields and make plants more nutritious.
“It’s a very substantial advance,” says Mohammed AlQuraishi, a systems biologist at Columbia University who has developed his own software for predicting protein structure. “It's something I simply didn't expect to happen nearly this rapidly. It's shocking, in a way.”
“This really is a big deal,” says David Baker, head of the Institute for Protein Design at the University of Washington and leader of the team behind Rosetta, a family of protein analysis tools. “It’s an amazing achievement, like what they did with Go.”
Astronomical numbers
Identifying a protein’s structure is very hard. For most proteins, researchers have the sequence of amino acids in the ribbon but not the contorted shape they fold into. And there are typically an astronomical number of possible shapes for each sequence. Researchers have been wrestling with the problem at least since the 1970s, when Christian Anfinsen won the Nobel prize for showing that sequences determined structure.
The launch of CASP in 1994 gave the field a boost. Every two years, the organizers release 100 or so amino acid sequences for proteins whose shapes have been identified in the lab but not yet made public. Dozens of teams from around the world then compete to find the correct way to fold them up using software. Many of the tools developed for CASP are already used by medical researchers. But progress was slow, with two decades of incremental advances failing to produce a shortcut to painstaking lab work.
CASP got the jolt it was looking for when DeepMind entered the competition in 2018 with its first version of AlphaFold. It still could not match the accuracy of a lab but it left other computational techniques in the dust. Researchers took note: soon many were adapting their own systems to work more like AlphaFold.
This year more than half of the entries use some form of deep learning, says Moult. The accuracy overall was higher as a result. Baker’s new system, called trRosetta, uses some of DeepMind’s ideas from 2018. But it still came a “very distant second,” he says.
In CASP, results are scored using what’s known as a global distance test (GDT), which measures on a scale from 0 to 100 how close a predicted structure is to the actual shape of a protein identified in lab experiments. The latest version of AlphaFold scored well for all proteins in the challenge. But it got a GDT score above 90 for around two thirds of them. Its GDT for the hardest proteins was 25 points higher than the next best team, says John Jumper, who heads up the AlphaFold team at DeepMind. In 2018 the lead was around six points.
A score above 90 means that any differences between the predicted structure and the actual structure could be down to experimental errors in the lab rather than a fault in the software. It could also mean that the predicted structure is a valid alternative configuration to the one identified in the lab, within the range of natural variation.
According to Jumper, there were four proteins in the competition that independent judges had not finished working on in the lab and AlphaFold’s predictions pointed them towards the correct structures.
AlQuraishi thought it would take researchers 10 years to get from AlphaFold’s 2018 results to this year’s. This is close to the physical limit for how accurate you can get, he says. “These structures are fundamentally floppy. It doesn’t make sense to talk about resolutions much below that.”
Puzzle pieces
AlphaFold builds on the work of hundreds of researchers around the world. DeepMind also drew on a wide range of expertise, putting together a team of biologists, physicists and computer scientists. Details of how it works will be released this week at the CASP conference and in a peer-reviewed article in a special issue of the journal Proteins next year. But we do know that it uses a form of attention network, a deep-learning technique that allows an AI to train by focusing on parts of a larger problem. Jumper compares the approach to assembling a jigsaw: it pieces together local chunks first before fitting these into a whole.
DeepMind trained AlphaFold on around 170,000 proteins taken from the protein data bank, a public repository of sequences and structures. It compared multiple sequences in the data bank and looked for pairs of amino acids that often end up close together in folded structures. It then uses this data to guess the distance between pairs of amino acids in structures that are not yet known. It is also able to assess how accurate these guesses are. Training took “a few weeks,” using computing power equivalent to between 100 and 200 GPUs.
Dame Janet Thornton at the European Bioinformatics Institute in Cambridge, UK, has been working on proteins for 50 years. “That’s really as long as this problem has been around,” she said in a press conference last week. “I was beginning to think it would not get solved in my lifetime.”
Many drugs are designed by simulating their 3D molecular structure and looking for ways to slot these molecules into target proteins. Of course, this can only be done if the structure of those proteins is known. This is the case for only a quarter of the roughly 20,000 human proteins, says Thornton. That leaves 15,000 untapped drug targets. “AlphaFold will open up a new area of research.”
DeepMind says it plans to study leishmaniasis, sleeping sickness, and malaria, all tropical diseases caused by parasites, because they are linked to lots of unknown protein structures.
One drawback of AlphaFold is that it is slow compared to rival techniques. AlQuraishi’s system, which uses an algorithm called a recurrent geometrical network (RGN), can find protein structures a million times faster—returning results in seconds rather than days. Its predictions are less accurate, but for some applications speed is more important, he says.
Researchers are now waiting to find out exactly how AlphaFold works. “Once they describe to the world how they do it then a thousand flowers will bloom,” says Baker. “People will be using it for all kinds of different things, things that we can't imagine now.”
Even a less accurate result would have been good news for people working on enzymes or bacteria, says AlQuraishi: “But we have something even better, with immediate relevance to pharmaceutical applications.”
Deep Dive
Artificial intelligence
How to opt out of Meta’s AI training
Your posts are a gold mine, especially as companies start to run out of AI training data.
Why does AI hallucinate?
The tendency to make things up is holding chatbots back. But that’s just what they do.
Apple is promising personalized AI in a private cloud. Here’s how that will work.
Apple’s first big salvo in the AI wars makes a bet that people will care about data privacy when automating tasks.
This AI-powered “black box” could make surgery safer
A new smart monitoring system could help doctors avoid mistakes—but it’s also alarming some surgeons and leading to sabotage.
Stay connected
Get the latest updates from
MIT Technology Review
Discover special offers, top stories, upcoming events, and more.